Artificial Intelligence
Artificial Intelligence Syllabus
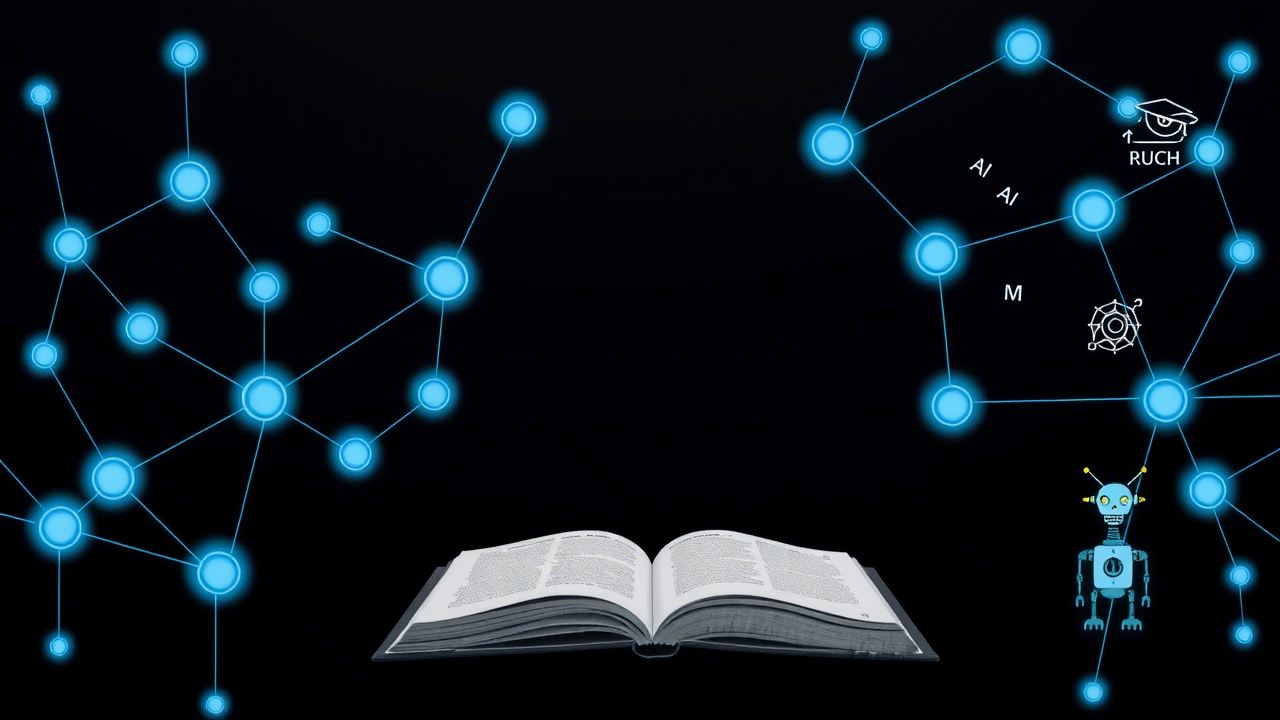
Introduction
Artificial Intelligence (AI) represents one of the most transformative technological fields of our time. This comprehensive course covers both classical AI approaches and cutting-edge techniques that power modern intelligent systems. Students will gain theoretical knowledge while building practical skills through integrated laboratory sessions, preparing them for careers in AI research, development, and application across various domains.
Course Objectives
- Understand AI fundamentals, techniques, and ethical implications.
- Design and implement intelligent agents, search algorithms, and machine learning models.
- Apply AI tools to real-world problems in robotics, healthcare, and NLP.
- Critically analyze societal impacts of AI systems.
Comprehensive Syllabus
1. Introduction to Artificial Intelligence
1.1 Fundamentals of AI
- 1.1.1 Definitions, Goals, and Approaches
- 1.1.2 Historical Evolution (1950s–Present)
- 1.1.3 AI Techniques: Symbolic vs. Subsymbolic Methods
1.2 Challenges in AI
- 1.2.1 Technical Barriers (Scalability, Data Quality)
- 1.2.2 Philosophical Debates (Consciousness, Ethics)
- 1.2.3 The Turing Test and Modern Alternatives (e.g., CAPTCHAs)
1.3 Applications
- 1.3.1 Healthcare (Diagnostic Systems)
- 1.3.2 Autonomous Systems (Self-Driving Cars)
- 1.3.3 Business Analytics (Fraud Detection)
- 1.3.4 AI for Social Good (Climate Modeling, Disaster Response)
1.4 Lab: Python/Prolog Basics for AI
- 1.4.1 Syntax and Data Structures
- 1.4.2 Introduction to Jupyter Notebooks and Prolog Environments
- 1.4.3 Setting up AI Development Environments (TensorFlow, PyTorch)
2. Intelligent Agents
2.1 Agent Architecture
- 2.1.1 Rational Agents vs. Omniscient Agents
- 2.1.2 PEAS Framework (Performance, Environment, Actuators, Sensors)
- 2.1.3 Agent Design Patterns and Architectures
2.2 Environment Types
- 2.2.1 Observable vs. Partially Observable
- 2.2.2 Deterministic vs. Stochastic
- 2.2.3 Episodic vs. Sequential
- 2.2.4 Static vs. Dynamic
- 2.2.5 Discrete vs. Continuous
2.3 Agent Types
- 2.3.1 Reflex Agents (Simple, Model-Based)
- 2.3.2 Goal-Based Agents
- 2.3.3 Utility-Based Agents
- 2.3.4 Learning Agents
- 2.3.5 Multi-Agent Systems
2.4 Lab: Agent Simulation
- 2.4.1 Implement a Vacuum-Cleaner Agent (Python)
- 2.4.2 PEAS Design for a Delivery Robot
- 2.4.3 Multi-Agent Coordination Simulation
3. Problem Solving by Searching
3.1 Uninformed Search
- 3.1.1 Breadth-First Search (BFS)
- 3.1.2 Depth-First Search (DFS)
- 3.1.3 Uniform Cost Search
- 3.1.4 Iterative Deepening
- 3.1.5 Complexity Analysis (Time/Space)
3.2 Informed Search
- 3.2.1 Greedy Best-First Search
- 3.2.2 A* Algorithm
- 3.2.3 Admissible Heuristics
- 3.2.4 Iterative Deepening A* (IDA*)
- 3.2.5 Memory-Bounded Search (SMA*, RBFS)
3.3 Adversarial Search
- 3.3.1 Minimax Algorithm
- 3.3.2 Alpha-Beta Pruning
- 3.3.3 Game Trees for Tic-Tac-Toe and Chess
- 3.3.4 Expectimax Search for Stochastic Games
- 3.3.5 Monte Carlo Tree Search (MCTS)
3.4 Lab: Search Algorithms
- 3.4.1 Implement BFS/DFS for Maze Solving
- 3.4.2 Build a Tic-Tac-Toe AI with Minimax
- 3.4.3 Develop a Pathfinding Algorithm with A*
- 3.4.4 MCTS Implementation for Simple Games
4. Knowledge Representation & Reasoning
4.1 Logic-Based Systems
- 4.1.1 Propositional Logic
- 4.1.2 First-Order Logic
- 4.1.3 Resolution and Inference Rules
- 4.1.4 Unification and Substitution
- 4.1.5 Theorem Proving
4.2 Structured Knowledge
- 4.2.1 Semantic Networks
- 4.2.2 Ontologies and Knowledge Graphs
- 4.2.3 Rule-Based Systems (Forward Chaining)
- 4.2.4 Rule-Based Systems (Backward Chaining)
- 4.2.5 Frames and Scripts
4.3 Probabilistic Reasoning
- 4.3.1 Bayesian Networks
- 4.3.2 Markov Models and Hidden Markov Models
- 4.3.3 Probabilistic Graphical Models
- 4.3.4 Exact and Approximate Inference
- 4.3.5 Decision Networks and Utility Theory
4.4 Lab: Knowledge Representation Systems
- 4.4.1 Prolog for Family Tree Relationships
- 4.4.2 Expert System for Medical Diagnosis
- 4.4.3 Building a Bayesian Network for Risk Assessment
- 4.4.4 Knowledge Graph Implementation
5. Machine Learning
5.1 Supervised Learning
- 5.1.1 Linear and Logistic Regression
- 5.1.2 Decision Trees (ID3, C4.5, CART)
- 5.1.3 Support Vector Machines
- 5.1.4 Neural Networks (Perceptrons, Backpropagation)
- 5.1.5 Ensemble Methods (Random Forests, Boosting)
5.2 Unsupervised Learning
- 5.2.1 K-Means Clustering
- 5.2.2 Hierarchical Clustering
- 5.2.3 PCA for Dimensionality Reduction
- 5.2.4 Association Rule Learning
- 5.2.5 Anomaly Detection
5.3 Deep Learning
- 5.3.1 Feedforward Neural Networks
- 5.3.2 Convolutional Neural Networks (CNNs)
- 5.3.3 Recurrent Neural Networks (RNNs)
- 5.3.4 Long Short-Term Memory (LSTM)
- 5.3.5 Transfer Learning and Fine-Tuning
5.4 Reinforcement Learning
- 5.4.1 Markov Decision Processes
- 5.4.2 Q-Learning and SARSA
- 5.4.3 Policy Gradient Methods
- 5.4.4 Deep Reinforcement Learning (DQN, A3C)
- 5.4.5 Multi-Agent Reinforcement Learning
5.5 Lab: Machine Learning Implementation
- 5.5.1 Train a Classifier on MNIST Dataset
- 5.5.2 Implement a Neural Network from Scratch
- 5.5.3 Transfer Learning with Pre-trained Models
- 5.5.4 Reinforcement Learning for Game Playing
- 5.5.5 ML Model Optimization and Deployment
6. Natural Language Processing (NLP)
6.1 Foundations
- 6.1.1 Tokenization and Word Embeddings
- 6.1.2 POS Tagging and Parsing
- 6.1.3 Named Entity Recognition
- 6.1.4 Sequence-to-Sequence Models
- 6.1.5 Attention Mechanisms
6.2 Advanced NLP Models
- 6.2.1 Transformer Architecture
- 6.2.2 BERT and Contextual Embeddings
- 6.2.3 GPT and Large Language Models
- 6.2.4 Zero-shot and Few-shot Learning
- 6.2.5 Multilingual Models
6.3 Applications
- 6.3.1 Sentiment Analysis
- 6.3.2 Machine Translation
- 6.3.3 Question Answering
- 6.3.4 Text Summarization
- 6.3.5 Conversational AI
6.4 Lab: NLP Tasks
- 6.4.1 Text Classification with HuggingFace
- 6.4.2 Fine-tuning BERT for Custom Tasks
- 6.4.3 Building a Text Summarization System
- 6.4.4 Chatbot Development (Rasa/Dialogflow)
- 6.4.5 Multilingual NLP Application
7. Generative AI
7.1 Foundations
- 7.1.1 Variational Autoencoders (VAEs)
- 7.1.2 Generative Adversarial Networks (GANs)
- 7.1.3 Diffusion Models
- 7.1.4 Autoregressive Models
- 7.1.5 Energy-Based Models
7.2 Applications
- 7.2.1 Image Generation (DALL-E, Stable Diffusion)
- 7.2.2 Text-to-Image Synthesis
- 7.2.3 Music and Audio Generation
- 7.2.4 Text Generation and Completion
- 7.2.5 Multimodal Generation
7.3 Lab: Generative Models
- 7.3.1 Building a Simple GAN
- 7.3.2 Fine-tuning Pre-trained Generative Models
- 7.3.3 Implementing a Text-to-Image System
- 7.3.4 Creating a Music Generation Application
- 7.3.5 Evaluating Generative Model Outputs
8. Expert Systems
8.1 Fundamentals of Expert Systems
- 8.1.1 Human Expert vs Expert System
- 8.1.2 Expert System Structure and Architecture
- 8.1.3 Characteristics of Expert Systems
- 8.1.4 Comparison with Traditional Software
- 8.1.5 Historical and Modern Expert Systems
8.2 Knowledge Engineering
- 8.2.1 Knowledge Acquisition Techniques
- 8.2.2 Knowledge Base Organization
- 8.2.3 Types of Knowledge (Declarative, Procedural)
- 8.2.4 Knowledge Representation for Expert Systems
- 8.2.5 Uncertainty Handling in Expert Systems
8.3 Inference Mechanisms
- 8.3.1 Forward Chaining
- 8.3.2 Backward Chaining
- 8.3.3 Mixed Chaining Strategies
- 8.3.4 Inference with Uncertainty
- 8.3.5 Explanation Facilities
8.4 Lab: Expert System Development
- 8.4.1 Tools for Expert System Development (CLIPS, Prolog)
- 8.4.2 Building a Simple Medical Diagnosis System
- 8.4.3 Knowledge Acquisition Exercise
- 8.4.4 Rule-Based System Implementation
- 8.4.5 Testing and Evaluating Expert Systems
9. Computer Vision
9.1 Fundamentals of Computer Vision
- 9.1.1 Image Formation and Representation
- 9.1.2 Digital Image Processing Basics
- 9.1.3 Color Models and Spaces
- 9.1.4 Feature Extraction Techniques
- 9.1.5 Historical Development of Computer Vision
9.2 Image Analysis
- 9.2.1 Edge Detection (Sobel, Canny)
- 9.2.2 Line and Shape Detection (Hough Transform)
- 9.2.3 Image Segmentation Methods
- 9.2.4 Texture Analysis
- 9.2.5 Motion Analysis and Optical Flow
9.3 Object Recognition
- 9.3.1 Template Matching
- 9.3.2 Feature-Based Recognition
- 9.3.3 Deep Learning for Object Detection
- 9.3.4 Instance vs. Semantic Segmentation
- 9.3.5 3D Scene Understanding
9.4 Advanced Topics
- 9.4.1 Face Detection and Recognition
- 9.4.2 Activity and Gesture Recognition
- 9.4.3 Visual SLAM (Simultaneous Localization and Mapping)
- 9.4.4 Augmented Reality Applications
- 9.4.5 Vision for Robotics
9.5 Lab: Computer Vision Applications
- 9.5.1 Image Processing with OpenCV
- 9.5.2 Implementing Basic Edge and Object Detectors
- 9.5.3 CNN-Based Object Recognition
- 9.5.4 Building a Face Recognition System
- 9.5.5 Real-time Computer Vision Application
10. Constraint Satisfaction Problems
10.1 Fundamentals of CSPs
- 10.1.1 Formal Definition and Examples
- 10.1.2 Constraint Types and Representations
- 10.1.3 Variables, Domains, and Constraints
- 10.1.4 Complexity Analysis of CSPs
- 10.1.5 Relationship to Search Problems
10.2 CSP Solving Techniques
- 10.2.1 Backtracking Search
- 10.2.2 Constraint Propagation
- 10.2.3 Arc Consistency Algorithms
- 10.2.4 Forward Checking
- 10.2.5 Minimum Remaining Values and Degree Heuristics
10.3 Advanced CSP Methods
- 10.3.1 Local Search for CSPs
- 10.3.2 Genetic Algorithms for CSPs
- 10.3.3 Distributed CSPs
- 10.3.4 Soft Constraints and Optimization
- 10.3.5 Temporal and Dynamic CSPs
10.4 Lab: CSP Applications
- 10.4.1 Implementing Cryptarithmetic Solvers
- 10.4.2 N-Queens Problem Implementation
- 10.4.3 Sudoku Solver Development
- 10.4.4 Scheduling Problem Applications
- 10.4.5 Building a CSP Framework
11. Ethics and Societal Impact
11.1 Ethical Frameworks
- 11.1.1 Consequentialism, Deontology, and Virtue Ethics
- 11.1.2 Bias Detection and Mitigation in ML Models
- 11.1.3 Fairness Metrics and Algorithmic Justice
- 11.1.4 Transparency and Explainability
- 11.1.5 Data Privacy and Consent (GDPR, CCPA)
11.2 Responsible AI Development
- 11.2.1 Human-Centered AI Design
- 11.2.2 Accountability and Governance
- 11.2.3 Safety and Alignment
- 11.2.4 Environmental Impact of AI Systems
- 11.2.5 AI in Critical Infrastructure
11.3 Case Studies
- 11.3.1 Autonomous Weapons Debate
- 11.3.2 Algorithmic Fairness in Hiring
- 11.3.3 AI in Healthcare Decision-Making
- 11.3.4 Surveillance Technologies
- 11.3.5 Synthetic Media and Misinformation
11.4 Lab: Ethics Workshop
- 11.4.1 Auditing a Dataset for Bias
- 11.4.2 Developing Explainable AI Methods
- 11.4.3 Design an Ethical AI Policy
- 11.4.4 Building a Fairness-Aware Model
- 11.4.5 Ethics Impact Assessment for AI Projects
12. Final Project
12.1 Guidelines
- 12.1.1 Topic Selection and Approval Process
- 12.1.2 Milestones: Proposal, Design, Prototype, Demo
- 12.1.3 Documentation and Presentation Requirements
- 12.1.4 Evaluation Criteria
12.2 Suggested Topics
- 12.2.1 AI for Climate Modeling and Sustainability
- 12.2.2 AI-Driven Educational Tools
- 12.2.3 Healthcare Predictive Analytics
- 12.2.4 Personalized Recommendation Systems
- 12.2.5 Computer Vision for Accessibility
- 12.2.6 Natural Language Understanding Applications
- 12.2.7 Robotics and Autonomous Systems
- 12.2.8 AI for Creative Expression
- 12.2.9 Expert System Development
- 12.2.10 CSP Applications in Real-world Problems
Prerequisites
- Data Structures and Algorithms
- Probability and Statistics
- Programming Proficiency (Python/Java)
- Basic Linear Algebra
- Calculus and Optimization Fundamentals
References
- Russell & Norvig, Artificial Intelligence: A Modern Approach (2020)
- Goodfellow, Bengio & Courville, Deep Learning (2016)
- Bishop, Pattern Recognition and Machine Learning (2006)
- Sutton & Barto, Reinforcement Learning: An Introduction (2018)
- Géron, Hands-On Machine Learning with Scikit-Learn, Keras, and TensorFlow (2022)
- Jurafsky & Martin, Speech and Language Processing (2021)
- E. Rich & K. Knight, Artificial Intelligence, McGraw-Hill, 1991
- E. Turban, Decision Support and Expert Systems, Macmillan, 1993
- G. Giarratano & G. Riley, Expert Systems: Principles and Programming, PWS Publishing, 1998
- Szeliski, Computer Vision: Algorithms and Applications, Springer, 2022
- Prince, Computer Vision: Models, Learning, and Inference, Cambridge University Press, 2012
- Online Resources: Coursera (Andrew Ng’s ML Course), TensorFlow/PyTorch Tutorials, HuggingFace Documentation
- Journal Articles: AI Magazine, Journal of Artificial Intelligence Research, Nature Machine Intelligence
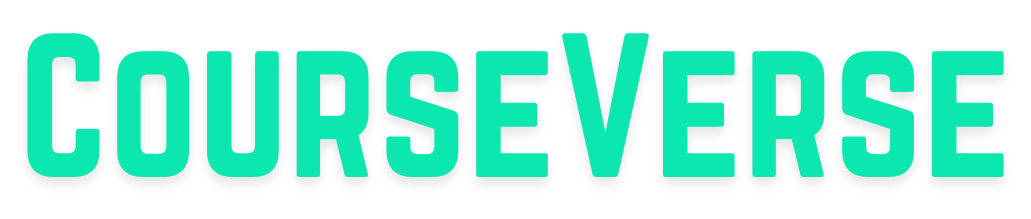
-
Fashion8 years ago
These ’90s fashion trends are making a comeback in 2017
-
Fashion8 years ago
According to Dior Couture, this taboo fashion accessory is back
-
Entertainment8 years ago
The old and New Edition cast comes together to perform
-
Business8 years ago
Uber and Lyft are finally available in all of New York State
-
Entertainment8 years ago
The final 6 ‘Game of Thrones’ episodes might feel like a full season
-
Entertainment8 years ago
‘Better Call Saul’ has been renewed for a fourth season
-
Politics8 years ago
Congress rolls out ‘Better Deal,’ new economic agenda
-
Entertainment8 years ago
Meet Superman’s grandfather in new trailer for Krypton